Registration is now closed. We are at full capacity with ~80 registered AI and Neuroscience enthusiasts. We are looking forward to seeing you in September.
Welcome to NEAT – Neuro-AI-Talks in Osnabrück – an event for EU-based research groups working at the intersection of neuroscience and artificial intelligence. The focus of NEAT is to foster connections and discussions among attendees, sharing new ideas, projects, and directions, as well as exploring potential collaborations. The event is aiming for a rather small group of attendees (approximately 70-80 people, invite only) to encourage an open and relaxed exchange. Each research group is welcome to join with 3-4 members and their PI. We look forward to welcoming you to Osnabrück in September.
Questions? Feel free to contact Katja Ruge at katja.ruge(at)uni-osnabrueck.de.
Important dates
Hotel reservation deadline: 31 July 2023
Welcome reception: 24 September 2023
Main event: 25 September 2023
Venue
![]() |
Hotels
Vienna House Remarque Walking distance to venue: 15 minutes Single room: 109 Eur/night per room Double room: 109 Eur/night per room Booking codeword: “neat 2023” Web: Remarque Hotel |
Walhalla Hotel Walking distance to venue: 18 minutes Single room: 94 Eur/night Double room: 104 Eur/night Booking codeword: “neat 2023” Web: Hotel Walhalla |
Main workshop dinner (September 25th)
Address: Weidenstraße 2, 49080 Osnabrück
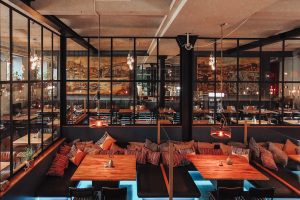
Web: https://portobar-restaurant.de/restaurant/
Due to the generous donations by our sponsors, the workshop dinner will be free of charge (registration required).
Keynote Speakers
Keynote 1:![]() Prof. Matthew Larkum Decoding the cortex: deep pyramidal insights into computation |
Keynote 2:![]() Prof. Mariya Toneva “Language modeling beyond language modeling.” |
Schedule
Sunday September 24, 2023 | |
17:00 – 19:00 | Welcome reception |
19:00 – 22:00 | Dinner matching Similar to CCN mind-matching, we plan to match scientists according to their research interests. Mind-matched groups will have joint dinner at local restaurants. |
Monday September 25, 2023 | |
08:30 – 09:00 | Registration |
09:00 – 09:15 | Welcome |
09:15 – 10:15 | Keynote 1 – Matthew Larkum |
Coffee Break | |
10:30 – 11:15 | Science Shuffle |
11:15 – 12:45 | Poster Session 1 |
Lunch | |
13:00 – 14:30 | Poster Session 2 |
14:30 – 15:30 | Debate Pods |
Coffee Break | |
16:00 – 17:00 | Keynote 2 – Mariya Toneva |
17:00 – 17:30 | Closing Remarks |
19:00 | Joint Dinner |
Posters
Katja Seeliger | Investigating the sensitivity of higher order visual areas with brain-optimization of common convolutional neural network architectures |
Sushrut Thorat | Characterising representation dynamics in recurrent neural networks for object recognition |
Jessica Thompson | Numerical reasoning with dual-stream neural networks. |
Alessandro T Gifford | A large and rich EEG dataset for modeling human visual object recognition. |
Ayu M I Gusti Bagus | High-Level Visual Cortex Representations Linearly Generalize Like Humans, Unlike current ANNs |
Adrien Doerig | Visuo-semantic transformation in the human brain and DNNs |
Kai Sandbrink | “How is control sensed and integrated into decision-making?” |
Johannes Singer | Revealing the locus and content of behaviorally relevant information about real-world scenes in human visual cortex. |
Laura Hansel | MorphOcc: Implicit Model for Representing Neuronal Morphologies |
Ahmed ElGazzar | Modelling neural dynamics with neural differential equations |
Agnessa Karapetian | Empirically identifying and computationally modelling the brain-behaviour relationship for human scene categorization. |
Siddharth Chaturvedi | Embodied Intelligence in Simple Dynamical Systems |
Sari Sadiya | Relating Artificial and Cognitive Representations |
Giacomo Aldegheri | Computational models of relational processing in human scene-selective cortex |
Micha Heilbron | Higher-level spatial prediction during natural scene perception in mouse visual cortex |
Maartje Koot | The Role of Predictive Dynamics in ANN Image Classification |
Joachim Bellet | Dynamic selectivity of visual features in macaque monkey prefrontal cortex: A comparative analysis with deep neural networks |
Noor Seijdel | Network depth improves scene segmentation: a critical test with computer generated images. |
Michaela Vystrcilova | Benchmarking system identification models of the retina. |
David Richter | What did you expect? Prediction error tuning in sensory cortex |
Farbod Nosrat Nezami | Time scale-plasticity learning rule for dendritic neuron model to achieve online time-invariant sequence processing |
Gabriele Merlin | Language models and brain alignment: beyond word-level semantics and prediction – Gabriele Merlin and Mariya Toneva |
Elaheh Akbarifathkouhi | Using CNNs to understand why we have an other-race effect |
Victoria Bosch | End-to-end topographic networks as models of cortical map formation and human visual behaviour: moving beyond convolutions |
Timo van Kerkoerle | Temporal dynamics of feature selectivity in neuronal populations in macaque monkey prefrontal cortex |
Philip Sulewski | The Active Visual Semantics Dataset: Understanding visual intelligence in action. |
Cliona O’Doherty | Time as a teacher – infant AI & fMRI |
Justus Hübotter | Spiking neural networks for robot control |
Sebastian Musslick | Augmenting EEG with Generative Adversarial Networks Enhances Brain Decoding Across Classifiers and Sample Sizes |
David-Elias Künstle | Psychophysical scaling with ordinal embedding methods |
Peter König | Improved spatial knowledge acquisition through sensory augmentation Improved spatial knowledge acquisition through sensory augmentation. |
Brett David Roads | Enriching ImageNet with Human Similarity Judgments and Psychological Embeddings. |
Johannes Mehrer | Topographic ANNs predict neural and behavioral responses to causal perturbations |
Shreya Kapoor | Perception of Mooney faces: Extreme Generalization through Inverse Rendering? |
Daniel Anthes | Diagnosing Catastrophe: Large Parts of Accuracy Loss in Continual Learning can be Accounted for by Readout Misalignment. |
Clemens G Bartnik | Human perception of navigational affordances in real-world environments |
Katharina Dobs | Using DNNs to understand why face perception works the way it does |
Dota Tianai Dong | How are Language and Vision Dynamically Integrated in the Brain During Naturalistic Movie Viewing |
Lea-Maria Schmitt | What recurrent dynamics underlie perceptual inference? |
Maria Eckstein | Predictive and Interpretable: Combining Artificial Neural Networks and Classic Cognitive Models to Understand Human Learning and Decision Making |
Pavithra Elumalai | Models for area V4 in free viewing macaques |
Amber Brands | Spatiotemporal adaptation through divisive normalization improves deep neural network recognition of objects in noise. |
Davide Cortinovis | The role of action-related properties in shaping the object space in the biological and artificial brain. |
Gabriele Merlin | Language models and brain alignment: beyond word-level semantics and prediction |
Niklas Müller | Investigating the Impact of High-Quality Natural Image Data for Training DCNNs; |
Bernhard Egger | ReWaRD: Retinal Waves for Pre-Training Artificial Neural Networks Mimicking Real Prenatal Development |
Event sponsors
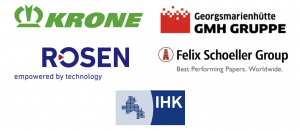